Author | Vanilla Editor | Xinyuan
Table tennis is indeed a top event at the Paris Olympics. Tonight, the Chinese men's team will face the Swedish team, which is expected to set off a surge in viewership and search trends. On the other side of the ocean, a U.S. research team is also preparing a big move with advanced technology.
On August 9, Zhi Dongxi reported that last night, Google DeepMind announced that its table tennis AI robot defeated human players, releasing multiple video demonstrations and providing a detailed introduction to the principles in a 29-page technical report.
It is said that this is the first Agent (intelligent agent) to reach the level of an amateur human in table tennis. Is Google going to create the AlphaGo of the table tennis world - "AlphaPingPong"?
This is not just a close match, but also a back-and-forth game. The robot not only easily masters skills such as smashes and switching between forehand and backhand, but also occasionally employs a "right adjust, left pressure" strategy, catching the opponent off guard.
Professional table tennis coach Barney J. Reed also praised it: "I am very surprised that the robot has reached an intermediate level, which even exceeds my expectations!"
Advertisement
In 29 matches against human players, the robot's average win rate reached 45%. Among them, the robot achieved a 100% overwhelming victory in the battle with novice players, won 55% of intermediate players, but lost all matches with advanced players.In post-match interviews, most of the players who competed against the robots found the experience fun and challenging. They didn't feel satisfied with just three games and wished to play another round!
Domestically, numerous universities and tech companies have also made breakthroughs in the research of table tennis AI robots. For instance, the "Xiao Qiu" robot from the University of Shanghai for Science and Technology recently played against Professor Yang Ming, the men's singles champion from Harbin Institute of Technology, attracting an audience of hundreds of thousands. "Xiao Qiu" set a Guinness World Record for the most consecutive human-machine table tennis rallies in 2021 with 6,241 hits.
At last year's Hangzhou Asian Games, Chuangyi Technology's self-developed "AI Xu Xin" table tennis robot faced off against Xu Xin himself, known as the "Golden Left Hand of the Chinese National Table Tennis Team." After the match, Xu Xin remarked, "The AI's hand movements are exactly the same as my own."
What about Google's table tennis AI robot? Let's explore its capabilities through demonstration videos and technical reports.
Paper link:
I. Won 13 out of 29 matches, with novice players being completely defeated
The hardware part of this table tennis robot is a 6-DOF ABB 1100 arm, mounted on two Festo linear gantry systems, capable of moving in a two-dimensional plane. The gantry system spans 4 meters across the table in the horizontal direction; it can move towards or away from the table in the longitudinal direction, with a length of 2 meters. A 3D-printed racket handle and a racket with short pimple rubber are installed on the robotic arm.▲ Table Tennis Robot Battles Professional Coach
To compete with humans, a robot must excel in basic skills such as returning the ball and smashing, as well as advanced skills like devising strategies and long-term planning to achieve goals.
The robot first trains in a simulated environment that accurately mimics the physical characteristics of a table tennis match. Once deployed into the real world, it collects performance data from playing against humans, thereby refining its skills in the simulation, forming a continuous feedback loop.
▲ Simulation Training
The system is also designed to adapt to the opponent's style by tracking the opponent's behavior and match style to adapt to different opponents, such as a tendency to return the ball to which side of the table. In this way, the robot can try different skills, monitor the success rate, and adjust its strategy in real-time.
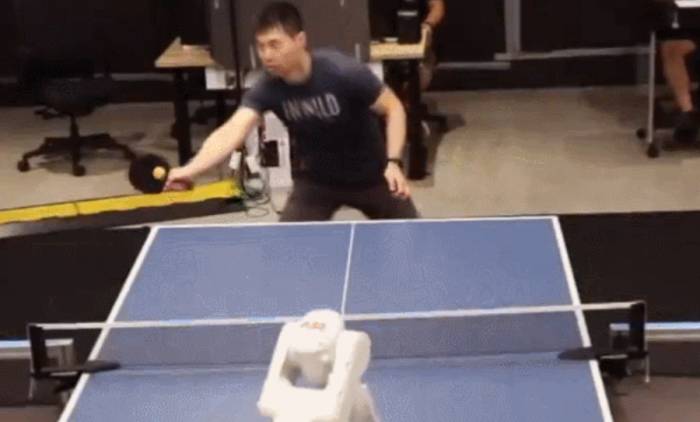
▲ Robot vs. Human
To evaluate the skill level of the robot Agent, 29 table tennis players of different skill levels competed against it. Based on a questionnaire survey of table tennis experience and scoring by professional coaches, these athletes were divided into beginners, intermediate, advanced, and super advanced levels.Among them, beginners and intermediate players have hardly ever received coaching guidance, and have hardly ever participated in competitions. Beginners usually have less than one year of experience and play less than once a month; while intermediate players have usually played for more than a year, playing once or several times a week.
Advanced players have all been playing for more than three years and have received coaching guidance. Super advanced players have played for an even longer time and have participated in more competitions.
Participant ability division
Each human player competes in a 3-game match against a robot, following the standard 11-point scoring system, but not adhering to the "best of three" rule. Instead, regardless of winning or losing, all 3 games are played. Since the robot cannot serve, some rules have been modified, and human players cannot score or lose points during serving.
In 29 matches, the robot won 13 games, with a win rate of 45%. Among them, the robot achieved a 100% win rate against beginner players, and a 55% win rate against intermediate players.
However, due to hardware and technical limitations, the robot is still unable to defeat advanced athletes, often being knocked down by a fast ball. Influencing factors such as reaction speed, camera sensing ability, rotation processing, and paddle rubber make it difficult to accurately model in simulation.
Robot Agent vs advanced players
Looking specifically at the scoring situation, the robot's average scoring rate against low, medium, high, and super high-level players is 72%, 50%, 34%, and 34%, respectively, which can be said to be "fifty-fifty" with intermediate players. Although the robot lost all matches to advanced players, in terms of each game, the robot still won 6-7% of the game situations.When facing novice and intermediate players, the robot always wins the first game with a win rate of 100%; then in the second game, the robot's win rate against intermediate players drops to 27%, and it rises back to 36% in the third game.
After the match, DeepMind conducted post-match interviews and analysis to find out that human players often need to adapt to the new environment in the first game; by the second game, they can identify some weaknesses of the robot and attack them specifically; but by the third game, the robot has been able to learn from the opponent's tactics and improve the win rate.
▲ Match situation
In the post-match interviews, most players said that playing against the robot was fun and challenging. They mentioned that the robot has dynamics and excitement, and provides an opportunity to balance high-speed performance and human comfort in the game. When asked if they would like to play with the robot again, more than 70% of the players said "very willing."
After the three competitive matches, players also had an optional free play session, up to 5 minutes. Players played an average of 4 minutes and 6 seconds with the robot.
▲ Player feedback
Some advanced players found weaknesses in the robot's strategy, such as its poor performance in spinning the ball, and they still enjoy the "man-machine confrontation." In the post-match interviews, they talked about its potential to become a more dynamic practice partner than a ball launcher.
Two, hard study of 14,000 pull shots, simulation + field training cycle positive feedbackTo achieve a human-level performance in table tennis, robots need to possess high-speed motion capabilities, precise control, and real-time decision-making abilities; moreover, table tennis matches are dynamically complex, involving rapid eye-hand coordination and high-level strategies.
To address these issues, DeepMind has proposed a new method, which mainly includes four technical contributions: a hierarchical and modular strategy architecture; zero-shot simulation to real technology, including an iterative method for defining a training task distribution based on the real-world; real-time adaptation to unknown opponents; and user research testing the model in a physical environment for actual matches with humans.
The hierarchical and modular strategy architecture is outlined as follows. The agent consists of an LLC (Low-Level Controller) and an HLC (High-Level Controller) that selects the most effective skills.
▲Overall Framework
The LLC is responsible for providing a set of skills for the HLC to deploy these skills in its strategy. The training of the LLC is divided into three steps: first, training two general basic strategies, corresponding to the forehand and backhand strokes; second, adjusting the training data combination before fine-tuning the new strategy by adding a reward function component; and finally, evaluating the new strategy and determining whether the strategy exhibits the required characteristics.
▲Low-Level Controller
Each low-level skill strategy in the LLC focuses on a specific aspect of table tennis, such as forehand topspin, backhand aiming, or forehand serving. In addition to training the strategy itself, this method also collects and stores information about the strengths, weaknesses, and limitations of each low-level skill, and the resulting skill descriptors provide the robot with important information about its capabilities and shortcomings.
The HLC is responsible for making strategic decisions, such as the position of the return, the speed of the stroke, and the level of risk undertaken. Currently, the HLC can only perform simple strategies and is a preliminary concept verification of the entire system.▲ High-Level Controller (HLC)
After each stroke, the HLC first selects a style strategy for the current stroke state to decide which Low-Level Controller (LLC) to use for the return. If it is a serve, it will attempt to categorize the spin into topspin and backspin, and choose the corresponding LLC; otherwise, it must determine which LLC performs best by finding the most similar ball state in the corresponding LLC skill table and obtaining return statistics.
Once a shortlist of candidate LLCs is generated, the HLC selects the final LLC through a weighted selection. The chosen LLC will be queried at a frequency of 50Hz to compare with the current ball state to determine the robot's actions.
To train the robot, DeepMind collected 40 minutes of human match data, as well as 480 different serves from the server, serving as the seed dataset for the initial state of table tennis, including information such as position, velocity, and spin. The system uses this dataset for practice and learning different skills, such as forehand topspin, backhand aiming, and returning serves.
▲ Dataset
The initial dataset contains 2,600 initial ball states, with an additional 900 serve data collected independently. By training on the dataset through simulation, evaluating in the real world, and iterating through the dataset with annotated evaluation data, DeepMind ultimately completed seven cycles of rally ball dataset and two cycles of serve dataset iterations within three months, resulting in 14,000 rally ball state data and 3,400 serve state data.III. Over 4 Years of Technical Accumulation, Netizens: Sell Me One
Google's DeepMind team has been researching table tennis robots for several years. For instance, in 2020, DeepMind proposed a model-free algorithm that could control the robot's joints at a frequency of 100Hz to return table tennis balls, achieving an 80% return rate in various serves.
▲ Google's Past Research on Table Tennis Robots
In July 2022, Google released the robot strategy reinforcement learning technology i-Sim2Real, which uses deep reinforcement learning to achieve high-speed, dynamic table tennis. It can interact with human players for over 4 minutes without interruption, hitting the ball 340 times.
▲ i-Sim2Real
At this time, the robot still looked a bit clumsy, only capable of moving left and right to hit the ball with a forehand.
In the case of the robot table tennis high-speed learning system in September last year, by integrating and optimizing the perception subsystem and high-speed low-latency robot controller technologies, the Google team has achieved autonomous training and evaluation on physical robots. At this stage, the robot was much more agile than in the previous phase.▲ Robot Table Tennis
The release of this Agent has also impressed many netizens.
Some netizens are already eager to take it home: "As an amateur table tennis enthusiast, I would be very happy to purchase one in the future."
▲ Netizen Comments
"Is this robot an athlete in this year's Paris Olympics?"
▲ Netizen Comments
There are also netizens "calling out the enemy from a distance," calling out to Tesla Optimus: "Your opponent is coming!"▲ Netizen Comments
There are also some skeptical voices emerging. For example, some netizens believe it is not versatile enough: "Can you ask it in natural language why it decided to take a certain action? Can you ask it to increase the intensity of the strike or change the strategy? If you can't make the robot versatile enough, then why? What is the biggest obstacle?"
▲ Netizen Comments
Google researchers say that the significance of this robot table tennis player goes far beyond the world of table tennis. Its underlying technology can be applied to various robotic tasks from manufacturing to healthcare, which require quick response and adaptation to unpredictable human behavior, and the potential application range is very broad.
Conclusion: From intelligence to physical strength, AI sweeps through competitive sports
For creating AI models capable of defeating human game players, DeepMind is no stranger. From AlphaGo, which defeated the world Go champion, to the all-around chess expert AlphaZero, DeepMind has proven the great potential of AI in board games. Although Google's table tennis robot has not yet reached the level of advanced players, it is likely to compete with top international players in the future through step-by-step technical iterations.
In fact, cutting-edge technologies such as AI and robots have already been applied in professional event training. As early as 2020, the Chinese Table Tennis Institute used AI ball-serving robots in training, with one robot capable of serving three players at the same time, and providing training of different levels for different groups of people. In addition to table tennis, AI-assisted training has also been used in the early preparation for several competitive events such as basketball, diving, sailing, and swimming, providing athletes with personalized and precise training guidance.